Skal alle have firmajulegaver?
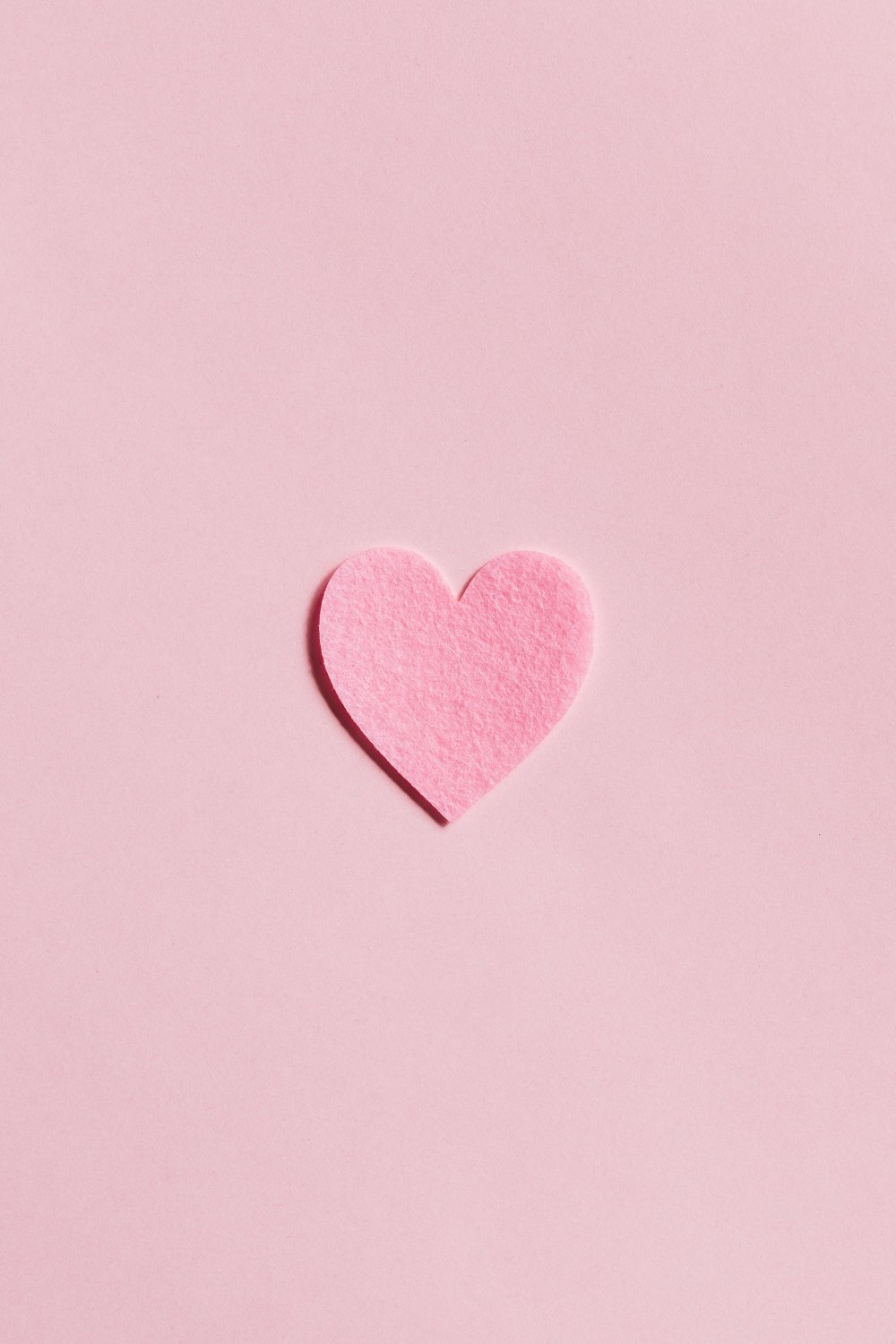
Hvis du virkelig agter at tilkendegive og vise din påskønnelse af alle i dit firma, så er der sådan set ingen vej udenom, at du tildeler alle firmajulegaver i foretagende, når denne beslutning er taget om, at du gerne vil give den slags gaver.
Der vil selvfølgelig være nogle begrænsninger, så dem vil vi starte med først.
Hvis du er leder af en afdeling af et større firma, så er det mere den her afdeling, du bør koncentrere dig om; hvis chefen for hele koncernen vil give alle ansatte firmajulegaver, må vedkommende selv sørge for dette.
Men man skal nok tjekke ind med direktøren for det hele først, for det kan jo give splid, hvis en underafdeling giver gaver til alle ansatte i den afdeling, mens de andre afdelinger bliver tilsidesat i den forbindelse.
Dog kan man godt sige, at hvis man har et søsterfirma i udlandet, eller hvor der som sådan intet samarbejde er andet end, at man har samme brand og nogle gange snakker sammen og sammenligner resultater, så behøver man ikke give gaver til dem også.
Retur til selve firmaet, hvor man vil give firmajulegaver, så er der ingen smalle steder her: alle skal have gaver.
En fordel er her, at man giver alle de ansatte de samme slags gaver, så man ikke føler, at der er nogen forskelsbehandling her; det er jo en anden måde at vise, at man værdsætter alles indsats, uanset hvad de laver her.
Dette gælder både, om man så taler om deltidsansatte, ansatte på nedsat arbejdstid, flextidsfolk, freelancere med videre.
Reelt set arbejder de forskelligt, men det afspejler sig også i lønnen, og det samme gælder ved den stilling, som man har.
Derfor bør disse firmajulegaver være af den samme slags, og der findes heldigvis mange forslag til at finde dem let og behændigt, uden man skal bruge for lang tid på at søge efter dette.